Jan Balewski
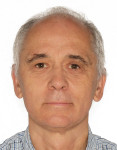
Biographical Sketch
Jan Balewski works at NERSC, exploring the utility of QuEra, IBM, IonQ, Quantinuum, and AQT@LBNL quantum processors for scientific computations. He has experience in machine learning HPC projects applied to cosmology and neuroscience data. Jan holds a Ph.D. in Physics from Jagiellonian University in Cracow, Poland. He has worked as a researcher at MIT's Laboratory for Nuclear Science (LNS) on the dark matter detection experiment DarkLight at JLAB and the polarized protons experiment STAR at RHIC/BNL.
Journal Articles
Jan Balewski, Mercy G. Amankwah, Roel Van Beeumen, E. Wes Bethel, Talita Perciano, Daan Camps, "Quantum-parallel vectorized data encodings and computations on trapped-ions and transmons QPUs", Nature Sci Rep 14, 3435 (2024), February 10, 2024,
E. Wes Bethel, Mercy G. Amankwah, Jan Balewski, Roel Van Beeumen, Talita Perciano, Daan Camps, "Quantum Computing and Visualization: A Disruptive Technological Change Ahead", IEEE Computer Graphics and Applications, vol. 43, no. 06, pp. 101-111, 2023., November 1, 2023,
Alexander Ladd, Jan Balewski, Kyung G. Kim, Kristofer Bouchard, Roy Ben-Shalom, "Scaling and Benchmarking an Evolutionary Algorithm for Constructing Biophysical Neuronal Models", Frontiers in Neuroinformatics, May 22, 2022,
William B Andreopoulos, Alexander M Geller, Miriam Lucke, Jan Balewski, Alicia Clum, Natalia Ivanova, Asaf Levy, "Deeplasmid: Deep learning accurately separates plasmids from bacterial chromosomes", Nucleic Acids Research, April 2, 2021,
Yosuke Oyama, Jan Balewski, and more, "The Case for Strong Scaling in Deep Learning: Training Large 3D CNNs With Hybrid Parallelism", IEEE Transactions on Parallel and Distributed Systems, December 1, 2020,
S. Lee, R. Corliss, I. Friščić, R. Alarcon, S. Aulenbacher, J. Balewski, S. Benson, J.C. Bernauer, J. Bessuille, J. Boyce, J. Coleman, D. Douglas, C.S. Epstein, P. Fisher, S. Frierson, M. Garçon, J. Grames, D. Hasell, C. Hernandez-Garcia, E. Ihloff, R. Johnston, K. Jordan, R. Kazimi, J. Kelsey, M. Kohl, A. Liyanage, M. McCaughan, R.G. Milner, P. Moran, J. Nazeer, D. Palumbo, M. Poelker, G. Randall, S.G. Steadman, C. Tennant, C. Tschalär, C. Vidal, C. Vogel, Y. Wang, S. Zhang,, "Design and operation of a windowless gas target internal to a solenoidal magnet for use with a megawatt electron beam", Nuclear Instruments and Methods in Physics Research Section A, September 21, 2019,
D Androić, J Balewski, and many more, "Precision measurement of the weak charge of the proton", Nature, May 9, 2018,
L. Adamczyk et al. (STAR Collaboration), "Measurement of Longitudinal Spin Asymmetries for Weak Boson Production in Polarized Proton-Proton Collisions at RHIC", Phys. Rev. Lett., August 13, 2014,
Conference Papers
Jan Balewski, Milan Kornjaca, Katherine Klymko, Siva Darbha, Mark R. Hirsbrunner, Pedro Lopes, Fangli Liu, Daan Camps, "Engineering quantum states with neutral atoms", IEEE International Conference on Quantum Computing and Engineering (QCE), April 5, 2024,
Jan Balewski, Daan Camps, Katherine Klymko, Andrew Tritt, "Efficient Quantum Counting and Quantum Content-Addressable Memory for DNA similarity", accepted at the IEEE23 quantum week conference, August 1, 2023,
We present QCAM, a quantum analogue of Content-Addressable Memory (CAM), useful for finding matches in two sequences of bit-strings. Our QCAM implementation takes advantage of Grover's search algorithm and proposes a highly-optimized quantum circuit implementation of the QCAM oracle. Our circuit construction uses the parallel uniformly controlled rotation gates, which were used in previous work to generate QBArt encodings. These circuits have a high degree of quantum parallelism which reduces their critical depth. The optimal number of repetitions of the Grover iterator used in QCAM depends on the number of true matches and hence is input dependent. We additionally propose a hardware-efficient implementation of the quantum counting algorithm (HEQC) that can infer the optimal number of Grover iterations from the measurement of a single observable. We demonstrate the QCAM application for computing the Jaccard similarity between two sets of k-mers obtained from two DNA sequences.
Adam Winick, Jan Balewski, Gang Huang, Yilun Xu,, "Predicting dynamics and measuring crosstalk with simultaneous Rabi experiments", APS March Meeting 2023, March 8, 2023,
"Co-design Center for Exascale Machine Learning Technologies (ExaLearn)", 2023 Exascale Computing Project Annual Meeting, December 22, 2022,
Jan Balewski, Zhenying Liu, Alexander Tsyplikhin, Manuel Lopez Roland, Kristofer Bouchard, "Time-series ML-regression on Graphcore IPU-M2000 and Nvidia A100", 2022 IEEE/ACM International Workshop on Performance Modeling, Benchmarking and Simulation of High Performance Computer Systems (PMBS), November 14, 2022, doi: 10.1109/PMBS56514.2022.00019
Yilun Xu, Gang Huang, Jan Balewski, Ravi K. Naik, Alexis Morvan, Brad Mitchell, Kasra Nowrouzi, David I. Santiago, Irfan Siddiqi, "Automatic Qubit Characterization and Gate Optimization with QubiC", ACM Transactions on Quantum Computing, April 13, 2022,
Grzegorz Muszynski, Prabhat, Jan Balewski, Karthik Kashinath, Michael Wehner, Vitaliy Kurlin, "Atmospheric Blocking Pattern Recognition in Global Climate Model Simulation Data", publication descriptionICPR 2020, May 5, 2021,
Steven Farrell, Murali Emani, Jacob Balma, Lukas Drescher, Aleksandr Drozd, Andreas Fink, Geoffrey Fox, David Kanter, Thorsten Kurth, Peter Mattson, Dawei Mu, Amit Ruhela, Kento Sato, Koichi Shirahata, Tsuguchika Tabaru, Aristeidis Tsaris, Jan Balewski, Ben Cumming, Takumi Danjo, Jens Domke, Takaaki Fukai, Naoto Fukumoto, Tatsuya Fukushi, Balazs Gerofi, Takumi Honda, Toshiyuki Imamura, Akihiko Kasagi, Kentaro Kawakami, Shuhei Kudo, Akiyoshi Kuroda, Maxime Martinasso, Satoshi Matsuoka, Henrique Mendonça, Kazuki Minami, Prabhat Ram, Takashi Sawada, Mallikarjun Shankar, Tom St. John, Akihiro Tabuchi, Venkatram Vishwanath, Mohamed Wahib, Masafumi Yamazaki, Junqi Yin, "MLPerf HPC: A Holistic Benchmark Suite for Scientific Machine Learning on HPC Systems", 2021 IEEE/ACM Workshop on Machine Learning in High Performance Computing Environments (MLHPC), February 13, 2021, doi: 10.1109/MLHPC54614.2021.00009
Yilun Xu, Gang Huang, Jan Balewski, Ravi Naik, Alexis Morvan, Bradley Mitchell, Kasra Nowrouzi, David I. Santiago, Irfan Siddiqi, "QubiC: An open source FPGA-based control and measurement system for superconducting quantum information processors", IEEE Transactions on Quantum Engineering, https://arxiv.org/abs/2101.00071, December 1, 2020,
M D Poat, J Lauret, J Porter, Jan Balewski, "STAR Data Production Workflow on HPC: Lessons Learned & Best Practices", 19th International Workshop on Advanced Computing and Analysis Techniques 2019, April 1, 2020,
Glenn K. Lockwood, Wucherl Yoo, Suren Byna, Nicholas J. Wright, Shane Snyder, Kevin Harms, Zachary Nault, Philip Carns, "UMAMI: a recipe for generating meaningful metrics through holistic I/O performance analysis", Proceedings of the 2nd Joint International Workshop on Parallel Data Storage & Data Intensive Scalable Computing Systems (PDSW-DISCS'17), Denver, CO, ACM, November 2017, 55-60, doi: 10.1145/3149393.3149395
I/O efficiency is essential to productivity in scientific computing, especially as many scientific domains become more data-intensive. Many characterization tools have been used to elucidate specific aspects of parallel I/O performance, but analyzing components of complex I/O subsystems in isolation fails to provide insight into critical questions: how do the I/O components interact, what are reasonable expectations for application performance, and what are the underlying causes of I/O performance problems? To address these questions while capitalizing on existing component-level characterization tools, we propose an approach that combines on-demand, modular synthesis of I/O characterization data into a unified monitoring and metrics interface (UMAMI) to provide a normalized, holistic view of I/O behavior.
We evaluate the feasibility of this approach by applying it to a month-long benchmarking study on two distinct large-scale computing platforms. We present three case studies that highlight the importance of analyzing application I/O performance in context with both contemporaneous and historical component metrics, and we provide new insights into the factors affecting I/O performance. By demonstrating the generality of our approach, we lay the groundwork for a production-grade framework for holistic I/O analysis.
Mustafa Mustafa, Jan Balewski, Jérôme Lauret, Jefferson Porter, Shane Canon, Lisa Gerhardt, Levente Hajdu2, Mark Lukascsyk, "STAR Data Reconstruction at NERSC/Cori, an adaptable Docker container approach for HPC", CHEP 2016, October 1, 2017,
Presentation/Talks
Yilun Xu, ..., J. Balewski, at al., FPGA-based Machine Learning for In-situ Qubit State Discrimination on QubiC, APS 2024 Spring meeting, May 23, 2024,
Jan Balewski, Programing Today's Quantum Computers, Seminar at LBNL Institute for Nuclear and Particle Astrophysics (INPA), February 1, 2024,
Jan Balewski, Mercy G. Amankwah, Roel Van Beeumen, E. Wes Bethel, Talita Perciano, Daan Camps,, "Quantum-parallel vectorized data encodings ", International Congress on Industrial and Applied Mathematics (ICIAM 2023), Tokyo, August 20, 2023,
Jan Balewski, Mercy G. Amankwah, Roel Van Beeumen, E. Wes Bethel, Talita Perciano, Daan Camps,, "Quantum-parallel vectorized data encodings ", Quantum Computer User Forum, on ORNL, July 17, 2023,
Jan Balewski, Managing dancing atoms, Quantum @ NERSC Training: Intro to QuEra, July 1, 2023,
User support models and experience using quantum hardware, NERSC seminar, April 17, 2023,
Jan Balewski, Gang Huang, Adam Winick, Yilun Xu, David I Santiago, Irfan Siddiqi, Ravi K Naik, Quantum processor crosstalk mitigation using QubiC controller aided by NERSC HPC, APS March Meeting 2022, March 16, 2022,
Gang Huang,Yilun Xu,Jan Balewski, QubiC - Qubits Control Systems at LBNL, Supercomputing Conference, SC20, November 11, 2020,
Jan Balewski, Interactive Cori Tutorial for users of LZ-experiment, February 24, 2017,
Tutorial w/ handouts. use of Shifter w/ image of chos=sl64 from PDSF Download the slides at https://docs.google.com/presentation/d/1Hh8vFE3ixxxiYTz9TgfljbUJcjmWUCNwzs-NugmLjSs/edit?usp=sharing