Physical Scientists Turn to Deep Learning to Improve Earth Systems Modeling
A Q&A with NERSC’s Karthik Kashinath
August 25, 2020
Contact: cscomms@lbl.gov
The role of deep learning in science is at a turning point, with weather, climate, and Earth systems modeling emerging as an exciting application area for physics-informed deep learning that can more effectively identify nonlinear relationships in large datasets, extract patterns, emulate complex physical processes, and build predictive models.
“Deep learning has had unprecedented success in some very challenging problems, but scientists want to understand exactly how these models work and why they do the things they do,” said Karthik Kashinath, a computer scientist and engineer in the Data & Analytics Services Group (DAS) at the National Energy Research Scientific Computing Center (NERSC) who has been deeply involved in NERSC’s research and education efforts in this area. “A key goal of deep learning for science is how do you design and train a neural network so that it can capture accurately the complexity of the processes it seeks to model, emulate, or predict, and we're developing ways to infuse physics and domain knowledge into these neural networks so that they obey the laws of nature and their results are explainable, robust, and trustworthy.”
We caught up with Kashinath following the “Artificial Intelligence for Earth System Science (AI4ESS) Summer School,” a week-long virtual event hosted in June by the National Center for Atmospheric Research (NCAR) and the University Corporation for Atmospheric Research (UCAR) that was attended by more than 2,400 researchers from around the world. Kashinath was involved in organizing and presenting at the event, along with David John Gagne and Rich Loft of NCAR. Much of Kashinath’s current research focuses on the application of deep learning methods to climate and Earth systems modeling.
How are deep learning methodologies being adopted in weather, climate, and Earth systems research?
In recent years we’ve seen a significant rise in the use of deep learning in science, not just in augmenting, enhancing or replacing existing methods, but also for discovering new science in physics, chemistry, biology, medicine, and more – discoveries that were nearly impossible with traditional statistical methods. We are now starting to see the same in the Earth sciences, with the number of publications in journals like Geophysical Research Letters and Nature Geoscience rising and scientific conferences now featuring entire tracks involving machine and deep learning.
What does deep learning bring to the table? It is extremely powerful in pattern recognition and discovering very complex nonlinear relationships that exist in large datasets, both of which are critical for developing models of Earth science systems. The key goal of a weather or climate modeler is to understand the ways in which processes in nature operate and to model them in an effective manner so we can predict the future of climate change and extreme weather events. Deep learning offers new methods for using existing data to understand how these processes operate and to develop models for them that are not only accurate and effective but also computationally much faster than traditional methods. Traditionally, climate and weather models solve large systems of coupled nonlinear partial differential equations, which is extremely computationally intensive. Deep learning is starting to augment, enhance, or even replace parts of these models with very efficient and fast physical process emulators. And that’s a significant step forward.
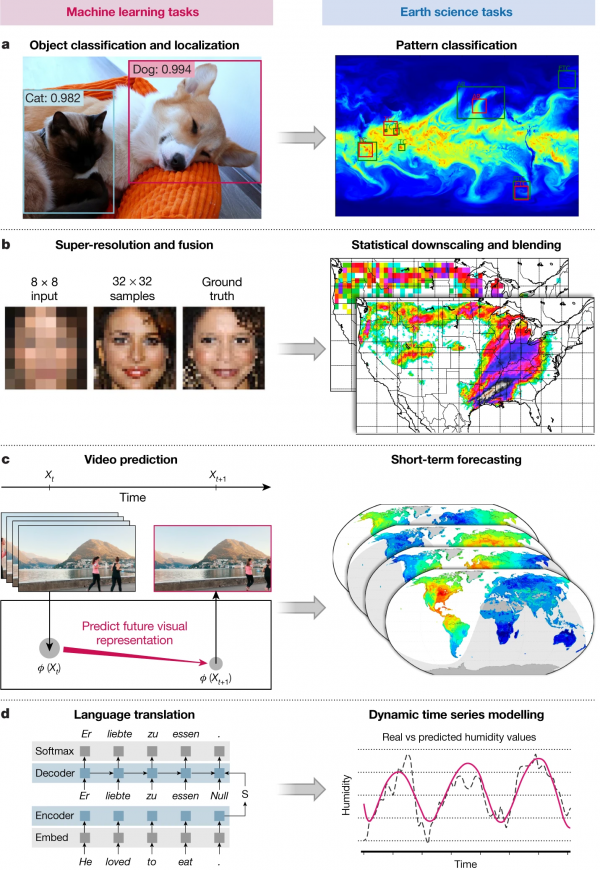
Examples of typical deep learning tasks (left panel) and the corresponding Earth system science problems that they can be applied to: a, Object recognition in images relates to detection of extreme weather patterns in climate data; b, Super-resolution relates to downscaling of climate data; c, Video prediction relates to forecasting of Earth system variables; d, Language translation relates to modeling of dynamic time series. (Source: Reichstein, M., Camps-Valls, G., Stevens, B. et al. Deep learning and process understanding for data-driven Earth system science. Nature 566, 195-04 [2019]. https://doi.org/10.1038/s41586-019-0912-1)
Pattern recognition is another area where deep learning is influencing Earth systems research. The DAS group at NERSC has been pushing hard on pattern recognition for detecting and tracking weather and climate patterns in large datasets. The 2018 Gordon Bell prize for exascale climate analytics using deep learning testifies to our contributions in that area. Given that we already have petabytes of climate data and that it is increasing at a crazy rate, it is physically impossible to sift through and recognize the key features and patterns using traditional statistical approaches. Deep learning offers very fast ways to mine that data and extract useful information such as extreme weather patterns.
A third area is downscaling; that is, given a low-resolution dataset, how do you produce very high-resolution data that is necessary for things like planning, especially on regional and local scales? Part of the grand challenge of climate science is how to build very high-resolution models that are accurate and produce data that we can reliably work with. One way to attack the problem is to say okay, we know these models are extremely expensive, and in the foreseeable future – even with computing getter faster and better – we’re really not going to be able to build reliable global climate models at a spatial resolution of 1 km or finer. So if we can create a deep learning model that takes low-resolution climate data and produces high-resolution data that is physically meaningful, reliable, and accurate – that is a game changer.
What is a grand challenge for deep learning applied to Earth system science?
I come from a background in fluid dynamics, where modeling turbulence is a long-standing grand challenge. A similar challenge in the atmospheric sciences is modeling clouds. All climate models have parameterizations – components in the climate model that describe how various physical processes behave and interact with each other. In the atmosphere that includes how clouds form, how radiation works, when and where precipitation happens, etc. Cloud modeling is also known to be the largest source of uncertainty in climate model projections, and for decades one of the big challenges has been how to reduce the uncertainty. Models have become much more complex and capture many more physical phenomena, but they still have large uncertainties in their predictions. So one area where deep learning could have a significant impact is to help us build better emulators of atmospheric processes like clouds, with the goal of reducing the uncertainties in predictions. That is a very concrete scientific goal.
As you look ahead, what are you most excited about in terms of the impact of deep learning on climate and Earth systems research?
The major pushback we’ve had from the scientific community is that neural networks are black boxes that are hard to understand and interpret, and scientists obviously would like to understand exactly how these neural networks work and why they do the things they do. So one thing I’m really excited about is developing better ways to interpret and understand these networks and incorporate the knowledge that we have about the physics of the Earth system into these models so they are more robust, reliable, trustworthy, interpretable, explainable, and transparent. The goal is to convince ourselves that these models are behaving in ways that respect the physics of nature, are effectively using the domain knowledge that we have, and are making predictions that we can trust. I was invited to submit a paper to Proceedings of the Royal Society on exactly this topic, “Physics-informed Deep Learning for Weather and Climate Modeling,” which is now under review.
I’m also excited about proving, in operation, that these deep learning models provide the computational speedup we claim they will provide when we embed them into a large climate or weather model. For example, the European Weather Forecasting Center has started to replace some parts of its weather forecasting model with machine and deep learning models, and they are already starting to see benefits. In the U.S., NCAR and the National Oceanic and Atmospheric Administration are also starting to replace parts of their climate and weather models with machine learning and deep learning models, and a number of academic and industry-based research groups are working on related projects. Chris Bretherton, one of the world’s leading climate scientists, heads a group at the University of Washington that is working to replace some of the complicated cloud processes in these large climate models with deep learning methods. So I’m looking forward to seeing their results in a year or two on speedup and performance.
What was the focus of the AI4ESS event, and why was it so well-attended?
The Artificial Intelligence for Earth System Science (AI4ESS) Summer School focused on how attendees can strengthen their background in statistics and machine learning, learn the fundamentals of deep learning and neural networks, and learn how to use these for challenging problems in the Earth system sciences. We had an overwhelming response to the school – it was supposed to be an in-person event in Boulder, Colo., with a capacity of 80 students. But once it went virtual, we had 2,400 attendees from 40 countries across the globe. It was live-streamed through UCAR and they tracked the daily log-ins.
There was great participation throughout the week. We had invited speakers every day – three lectures a day, so 15 lectures over the week – with experts from machine learning, deep learning, and the Earth sciences. Each day there was also a panel discussion for 30 minutes over lunch, and for me, these were super exciting because all of these experts were discussing and debating about the challenges and opportunities of using machine learning and deep learning for Earth system science. The school also held a week-long hackathon, where teams of six each chose a project from six different problems to work on for the week. About 500 people participated in the hackathon, with a lot of collaboration and interaction, including individual Slack channels for each of the hackathon teams. There were also Slack channels for the entire week of the summer school on various things: lecture-related Q&As, hackathon challenge problems, technical tips and tricks in machine learning and deep learning, etc. So there was a lot of Slack activity going on, with people exchanging ideas, sharing results, and so forth.
Why is everyone so keen on learning this stuff? I think the community, especially the younger scientists, see that deep learning can be a game changer in science and they don’t want to be left behind. They believe that it is going to be mainstream soon and that it is going to be essential for doing science. That's the main motivator. So AI4ESS focused on teaching the fundamentals and laying the groundwork for them to begin applying machine and deep learning successfully to their research.
Located at Lawrence Berkeley National Laboratory, NERSC is a DOE Office of Science User Facility.
About NERSC and Berkeley Lab
The National Energy Research Scientific Computing Center (NERSC) is a U.S. Department of Energy Office of Science User Facility that serves as the primary high performance computing center for scientific research sponsored by the Office of Science. Located at Lawrence Berkeley National Laboratory, NERSC serves almost 10,000 scientists at national laboratories and universities researching a wide range of problems in climate, fusion energy, materials science, physics, chemistry, computational biology, and other disciplines. Berkeley Lab is a DOE national laboratory located in Berkeley, California. It conducts unclassified scientific research and is managed by the University of California for the U.S. Department of Energy. »Learn more about computing sciences at Berkeley Lab.